In 2022, the introduction of generative AI tools like ChatGPT, Gemini, and Dall-E marked a significant technological leap as these models processed complex queries to generate contextually relevant content.
According to UserInterview's 2023 survey, 77% of UX professionals reported using AI in their research workflow. As the capabilities of generative AI continue to expand, its potential to streamline and enhance UX research processes becomes increasingly apparent. New AI tools for research are available in the market and existing UX research tools, like Hubble, are adding AI capabilities to their platform.
In this article, we explore how AI-powered tools are currently helping streamline research and introduce some popular AI-powered tools for user research. We also introduce some best practices and potential pitfalls for using AI for user research.
Whether you're a product manager, UX designer or researcher, these tools can help you automate research and design process, uncover user insights, and ultimately increase your research ROI.
The Role of AI in UX Research (How AI can help)
Traditionally, user research is time-consuming and labor-intensive as it involves manual processes from setting up a research plan, recruiting and scheduling participants, and conducting interviews and usability tests to analyzing qualitative data.
Artificial intelligence is providing new ways to simplify the process, allowing product teams more time and space to focus on high-level strategy and interpreting key insights.
Overall, automation yields rapid turnaround, allowing UX designers to iterate more quickly, UX researchers to test more frequently, and so on.
The result is a more agile research process that can keep pace with the fast-moving demands of product development, ultimately leading to quicker, more informed decisions.
Automated transcription
Transcription services and features greatly help expedite the data cleaning and analysis step in user research by automatically converting spoken language from recordings and user interviews into written text. With increased accuracy and speed, automated transcription allows product teams to focus on analyzing the content rather than manually transcribing recordings.
Additionally, AI-driven transcription services often include features like speaker identification and time stamping, which further streamline the process of reviewing and organizing qualitative data.
Some tools also have sentiment analysis features to analyze user sentiment, suggesting positive or negative comments in the transcription. While automated transcriptions may occasionally require manual editing for accuracy, they offer a fast and efficient way to document user data.
Initial data analysis on large volume of data
One of the most significant contributions of AI in UX research is its ability to quickly analyze large volumes of data. AI tools can sift through hundreds of user feedback and numerical data to identify trends and insights that might take hours for human to process.
Whether its hundreds of survey results or paragraphs of interview transcriptions, analyzing large data is daunting to begin with. Use AI as a first pass to process large volume of data for identifying patterns or descriptive statistics.
Brainstorming and ideation
AI can be a useful for generating ideas and brainstorming in user research. It can suggest new concepts or features that align with the prompts that are provided.
These AI-driven suggestions can serve as a springboard for brainstorming sessions, helping teams explore new directions they might not have considered otherwise.
Automating manual tasks
AI is effective with automating repetitive and time-consuming tasks that traditionally burden product teams. For instance, AI tools can transcribe interviews, and clean and analyze research data for preliminary results.
Automation in various aspects reduce the workload on product team members while minimizing potential human error.
Automated insights and reporting
AI tools can automatically generate reports and insights from user data, saving product team members time and effort. With advanced NLP, AI tools have better capabilities to process textual data than before.
Even though it needs human oversight to validate the results, these tools can highlight key insights and findings. Regardless of the validity of the generated reports, such automated features can help UX researchers save time.
So will AI replace UX designers and UX researchers?
The short answer is no; it is a useful assistant given the current state of AI.
AI is undeniably transforming UX research by expediting and streamlining various aspects of the process, but it’s important to recognize that it won’t replace the human element.
AI excels at handling large quantitative data, automating repetitive tasks, and even uncovering patterns that might be missed by the human eye. However, when it comes to understanding the nuanced, qualitative aspects of user behavior and motivations, human skills and expertise remain irreplaceable.
Qualitative research involves interpreting subtle cues, emotions, and context that AI, despite its advancements, cannot fully grasp. For example, during in-depth interviews, UX researchers pick up on non-verbal cues, tone of voice, and the subtleties of a user’s experiences that AI might overlook. This human ability to empathize and connect with users on a deeper level is essential for truly understanding user behavior and creating user-centered designs.
Interpreting insights generated from AI tool requires a human touch. AI can suggest trends or correlations, but it takes UX professionals to discern whether these findings are relevant and how they should be applied within the broader context of the project. Human oversight also helps ensure that the insights are actionable, ethical, and aligned with the business goals.
Overview of best AI tools for UX research
We've curated a list of tools that use AI below:
The numbering is not indicative of ranking. It is simply for listing out the various collection of tools. Each tool has its strength and use with AI features.
1. Hubble
Hubble is a unified research tool that streamlines participant recruiting, study building, and data analysis. It offers in-product surveys to collect contextual user feedback from realtime users engaging with your product at specified touchpoints.
Its unmoderated study feature supports prototype and usability testing with Figma integration, live-site testing, card sorting, and more to design studies specifically to your research needs.
.png)
🤖 Key AI feature(s):
- AI-powered summary to initialize qualitative data analysis
- Advanced visualization for intuitive data analysis
- Hubble's AI features are all available at free tier
➕ Pros:
- In-product surveys for live contextual user feedback
- Figma integration for easy prototype testing
- Access to 3 million pool of high quality participants in partnership with Respondent
- 50+ research and product development related templates to kickstart your project
- Dedicated customer support team and Slack community
➖ Cons:
- Manual support for moderated sessions. Unlike unmoderated studies, requests for moderated sessions are not automated.
- Lacks support for Sketch and InVision
"Anyone in product would know that the more you get in front of your customers, it’s only going to benefit you whether that’s a positive or negative feedback from them. And Hubble makes it easier. So the sooner you try out Hubble, the better."
Minhtri Chu
Head of Product at Doorvest
2. Generative AI (ChatGPT, Gemini, Claude)
ChatGPT, Gemini, and Claude are different NLP models-based generative AI tools developed by Open AI, Google, and Anthropic. They output content based on text prompts. While it is not specifically built for user research, you can specify instructions and with prompt engineering, you can tailor results. While the outputs and performance vary by products and their models, these tools are easily accessible and free to use.

🤖 Key AI feature(s):
- Extensive content generation
- Serves as many base model/API for other AI-powered UX research tools
➕ Pros:
- Great for getting inspirations for brainstorming, ideation, and study questions
- Free powerful assistance for your daily workflow
➖ Cons:
- Not specifically built for research and lacks context of study or data unless you provide instructions
- May require some practice on prompt engineering to tailor results
- Token-based limit on the content generated
- Limit on feeding in user data for data synthesis
3. Qualtrics
Qualtrics is a powerful survey software, boasting advanced options for survey customization, advanced machine learning based analytics, and reporting features. While it is popularly used as a survey platform in user research, it also includes experience management system.
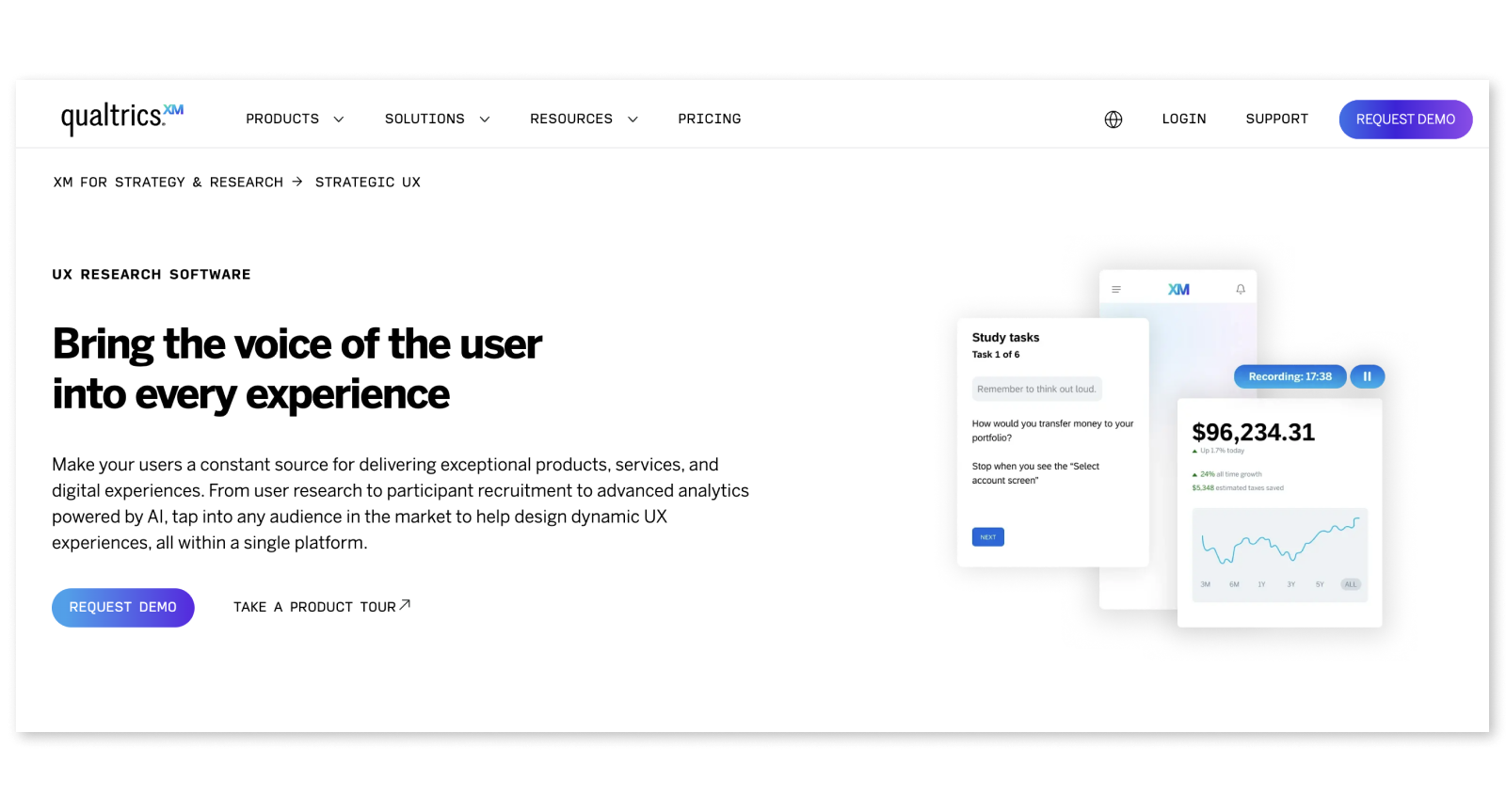
🤖 Key AI feature(s):
- Advanced machine learning model to support analytics of survey responses
- Transcription service
➕ Pros:
- Powerful survey tool with various kinds of question types and formats
- Fully customizable survey with its API
- Great survey distribution and reporting features
➖ Cons:
- Various features are available but come at a price
- Extensive set of features make it difficult to create simple surveys
4. QoQo
QoQo, powered by OpenAI (creator of ChatGPT), is a AI tool that is built for UX design and research: It helps create user journeys, user personas, and more. It is still in its beta phase, only accessible via Figma as a plugin. It is a great AI assistant specifically for UX designers and professionals actively using Figma.

🤖 Key AI feature(s):
- Figma plugin great for UX designers
- Generating user personas and user stories with highlights of user goals, needs, and pain points
- Create user journey maps
- Extensive prompt-based content generation for designers
➕ Pros:
- Works directly in Figma
- Create artifact and deliverables based on prompt
- Cheap price (~$7/month) to get started
➖ Cons:
- Still in its beta phase and only accessible via Figma
- Generating artifacts based on AI requires careful validation
5. UserTesting
UserTesting is a powerful user research tool that provides moderated and unmoderated study features. It is widely used for its extensive unmoderated study feature that supports video and screen recording, automatic transcription, and sentiment analysis.
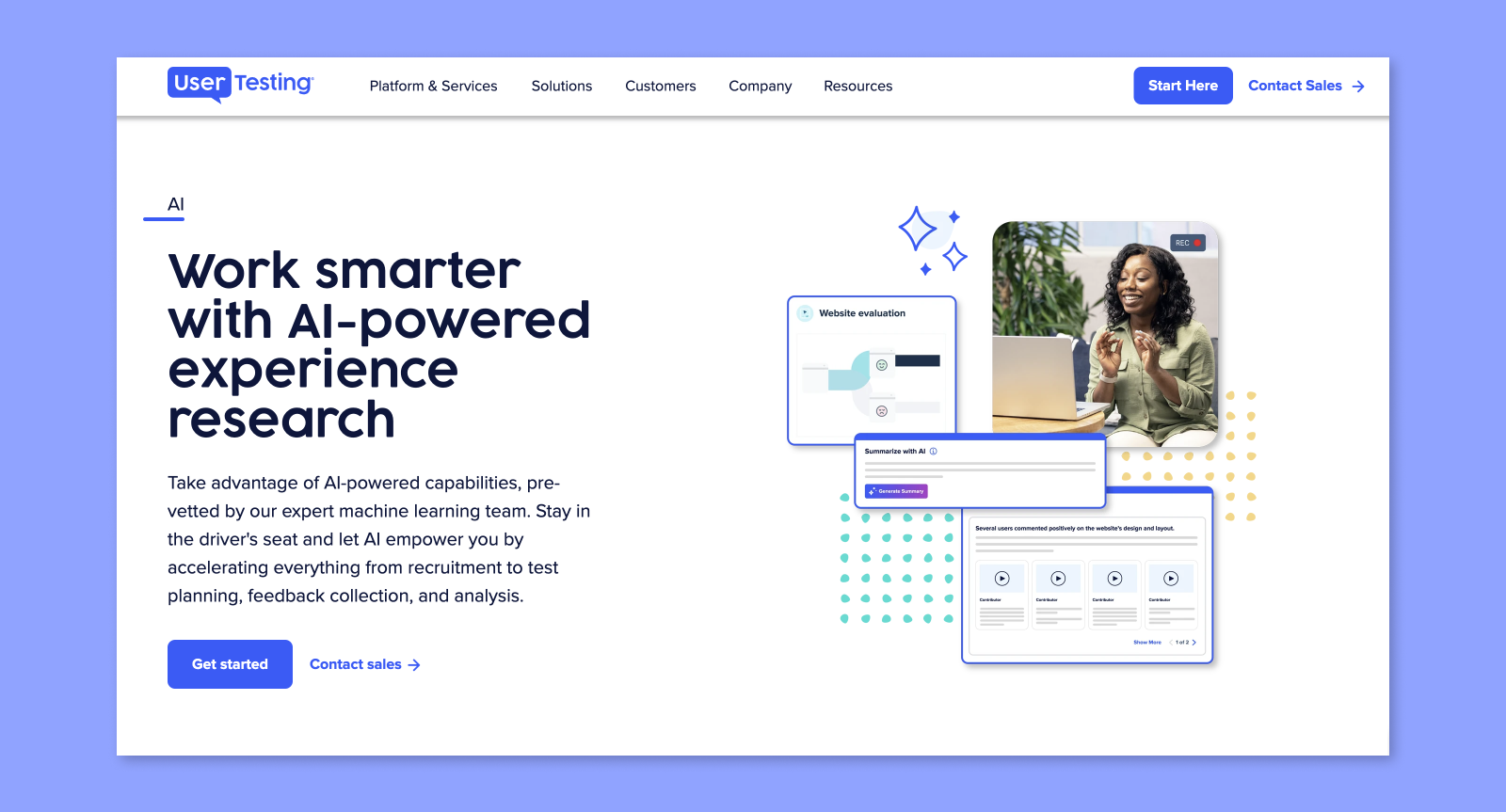
🤖 Key AI feature(s):
- Sentiment analysis on video transcription
- AI summaries and insights generation
➕ Pros:
- Extensive features for unmoderated testing and quick 5 minute testing
- Helpful video transcription and reviewing feature
➖ Cons:
- Inaccurate or unhelpful sentiment analysis
- While it offers its recruitment panel, the participant quality has been known to be fairly poor compared to User Interviews and Respondent
6. Maze
Maze is a user research platform that supports unmoderated and moderated user testing. It offers participant recruiting and reporting along with various user testing options, such as card sorting, tree testing, and etc.
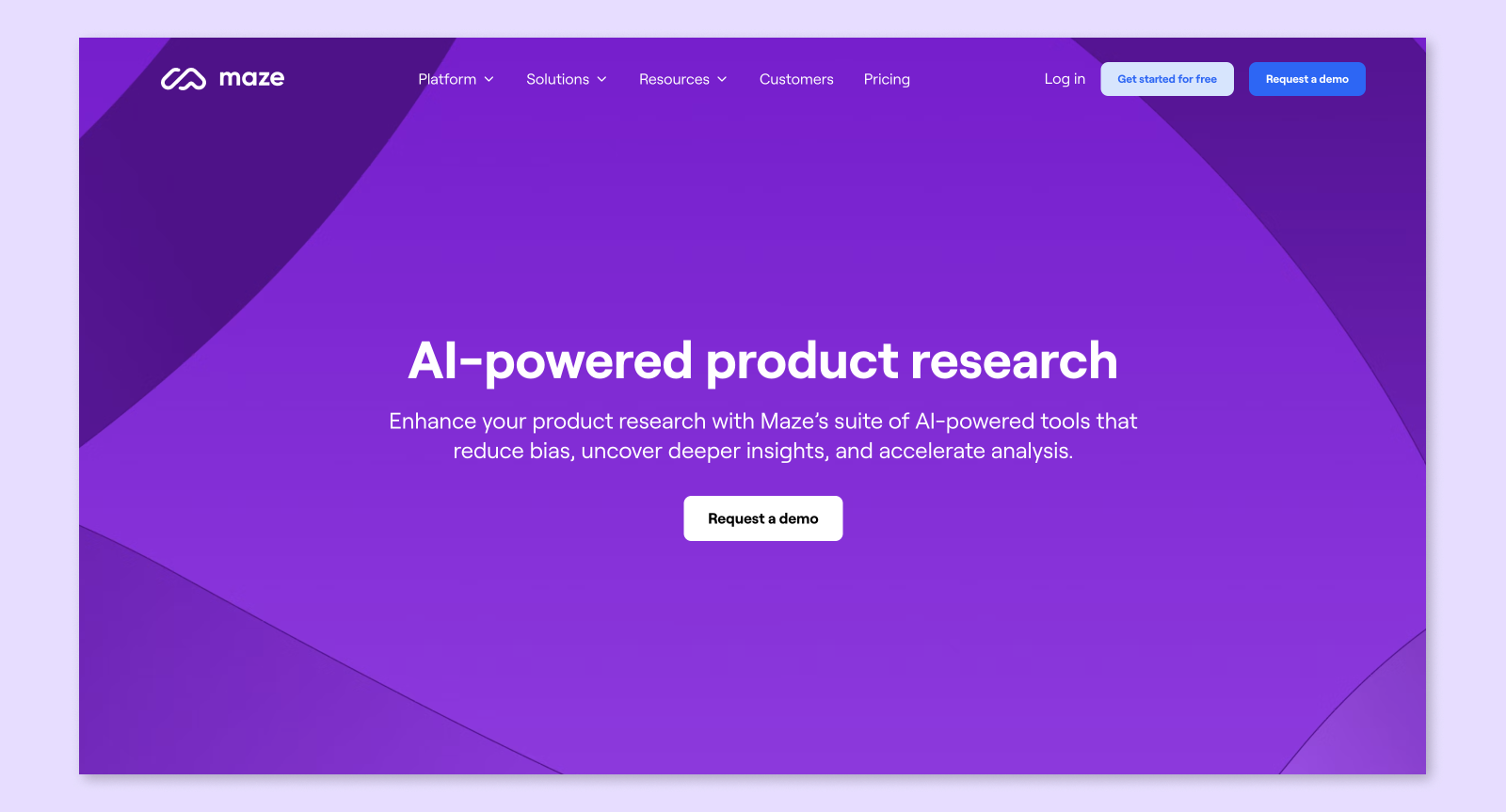
🤖 Key AI feature(s):
- AI insights and key highlights
- AI to proofread questions and generate contextual follow-up questions
➕ Pros:
- Offers various user testing options to suit your research needs
➖ Cons:
- Insights from AI require validation
- While it supports its own participant panel, its quality is unsure
7. Notably
Notably is a user research tool primarily for research repositories with AI-powered data coding, insights, and summary. Repository tool is mainly used to upload videos of user interview or focus groups, and help you leverage artificial intelligence to process data.
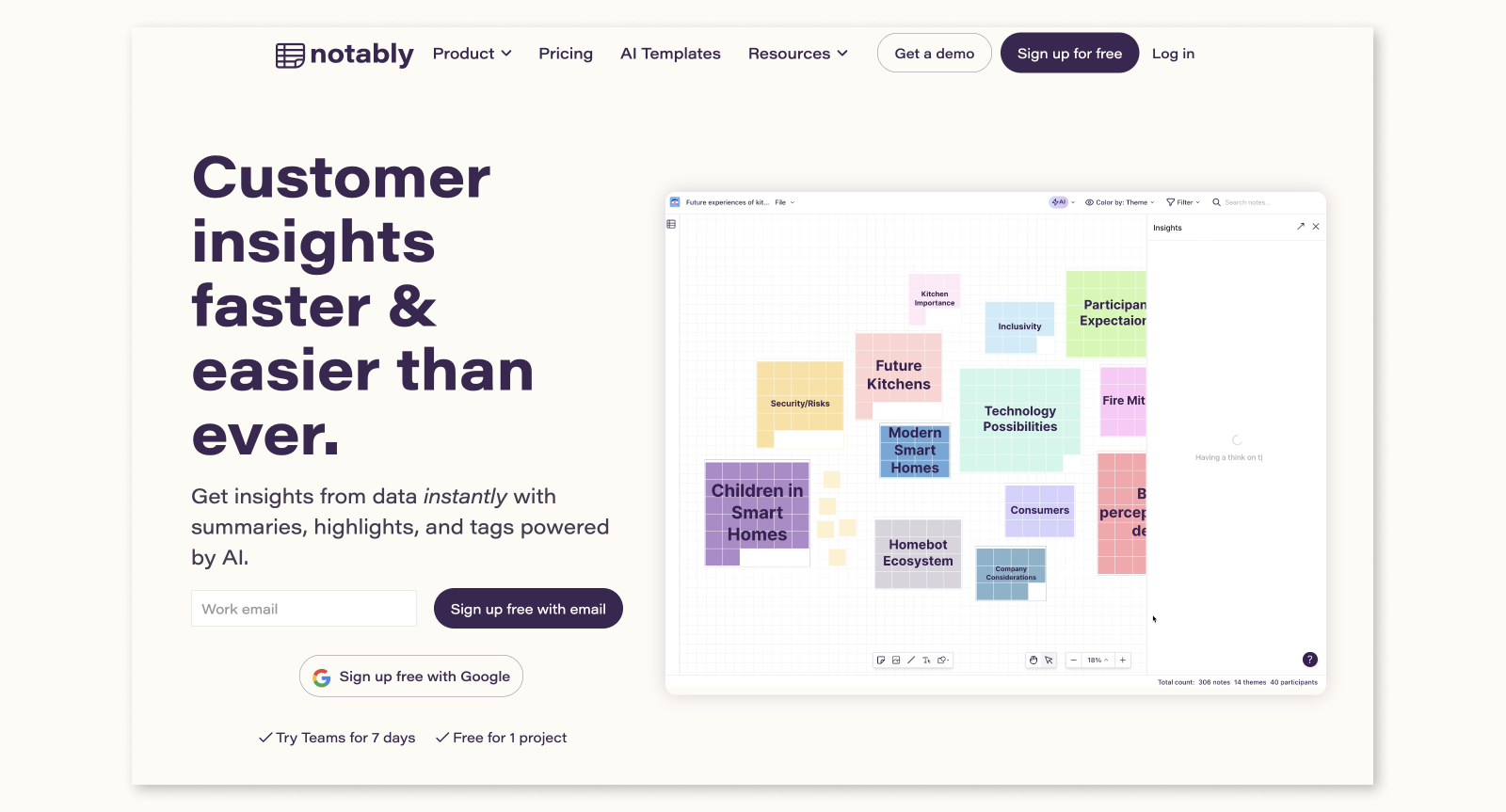
🤖 Key AI feature(s):
- Transcription for user interview data
- AI summaries, insights, and cluster analysis
➕ Pros:
- AI-powered research repositories
- Has whiteboard feature to visually organize data
➖ Cons:
- Like other prompt-based features, may require practice for prompt engineering
- Any insights from AI require validation
8. Looppanel
Looppanel is an AI powered research analysis tool built to facilitate with automatic transcription and note-taking. Consider it as a research assistant that helps you take notes while observing the sessions, organizing the results, and having a go-to-place for your research data collection.

🤖 Key AI feature(s):
- Automated note-taking and video transcription
- Repository management with AI feature
- Automated tagging on data
➕ Pros:
- AI note-taking and video transcription effective for processing qualitative data
➖ Cons:
- Is a tool specialized for research repository
9. Userdoc
UserDoc is a AI-powered tool for generating user stories, acceptance criteria, and persona (similar to QoQo) that can be used during project planning process to gauge the scope of each project.

🤖 Key AI feature(s):
- Generate user stories and persona
➕ Pros:
- Useful tool for the entire product teams for planning and scoping project early on
➖ Cons:
- Does not directly support any UX research methods or tool
10. Hotjar
Hotjar is a powerful web analytics tool that is adding new AI capabilities like AI-powered survey creation that creates relevant questions based on research questions.
Hotjar specializes in website analytics, heatmaps, session recordings, and surveys to visualize how your users interact with your product or websites.
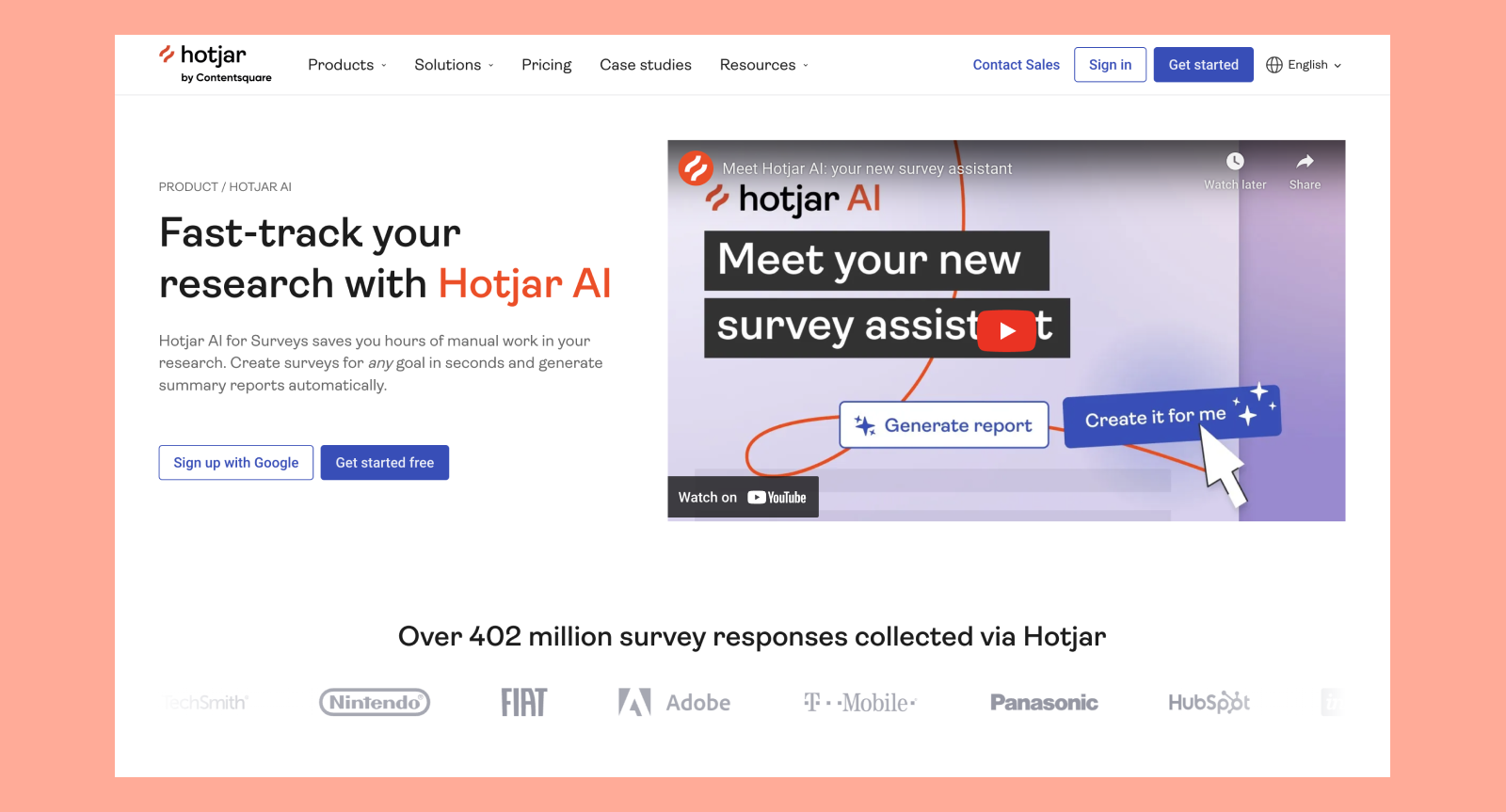
🤖 Key AI feature(s):
- Step-by-step AI-powered survey creation relevant to your research questions
➕ Pros:
- Specializes in survey and web analytics to capture user behavior
- AI-powered study creation saves time and resources
➖ Cons:
- Requires human oversight to guardrail results generated from AI
11. Notion
Notion is already a popular tool offering a centralized platform to organize, document, and collaborate on projects. You can create, manage notes, transcripts, research plans, and more all in one place.
If your team is already using Notion for research repository, managing data or participant recruitment, consider using its Notion AI feature that will help you analyze data and meeting notes, provide next steps, and create summaries.
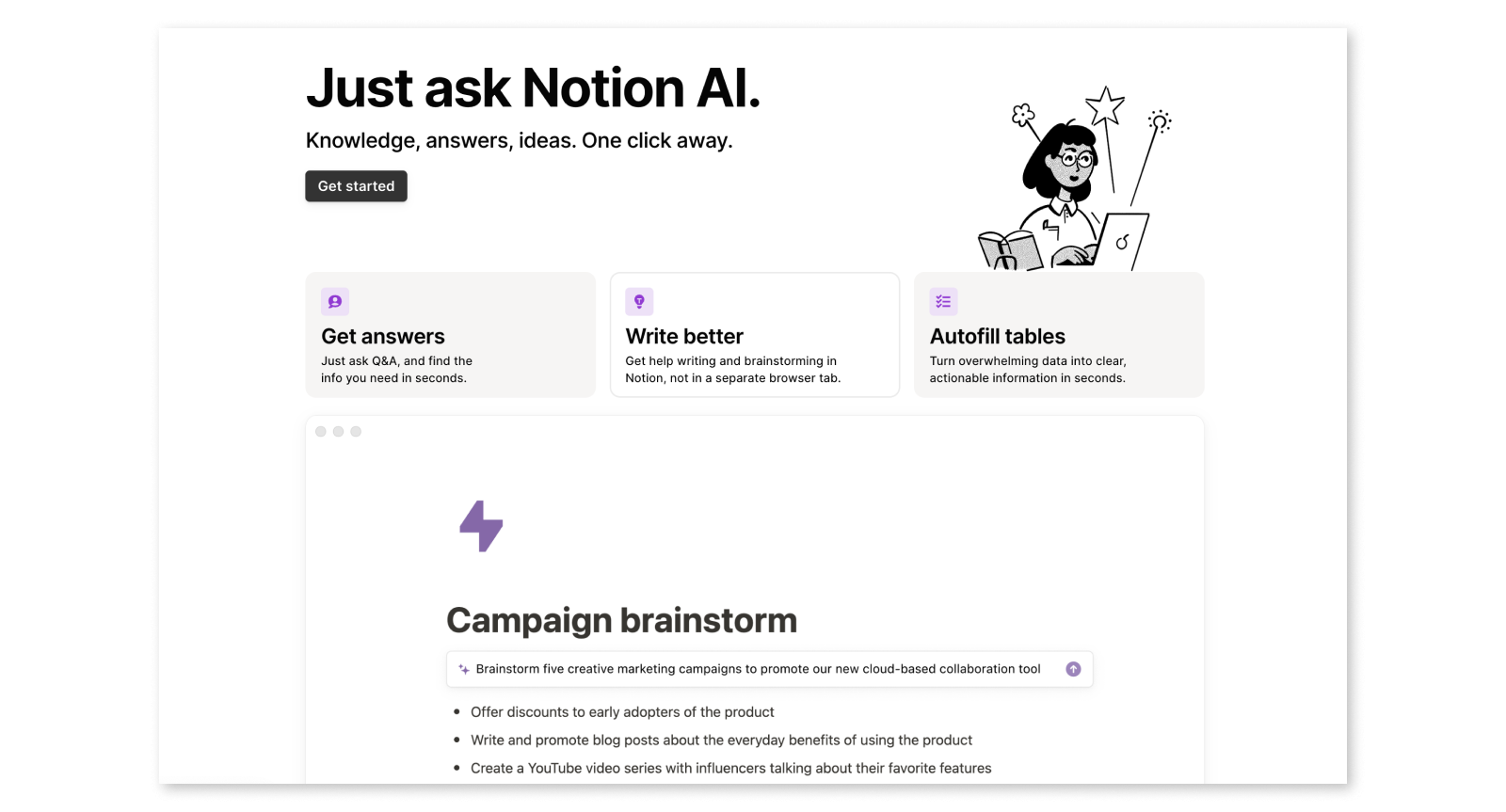
🤖 Key AI feature(s):
- AI analysis on meeting notes, creating summaries and next steps
- AI note-taking
➕ Pros:
- Popular tool with flexible data structure and easy to use
- Great for research repository and managing documentation
➖ Cons:
- Is a tool specifically for managing documentation
Selecting the right AI tool for your UX research
With new AI tools for user research emerging and existing software adding AI capabilities, there are numerous tools with AI capabilities to choose from. Assess tools based on their features, user reviews, compatibility with your existing systems, and how certain AI features could integrate with your user UX research and design process.
1. Functionality
The tool should offer features that match your specific research needs. Whether you require automated data analysis, sentiment analysis, or AI powered user testing capabilities, make sure the tool provides these functionalities. Look for tools that excel in your areas of needs, whether they provide different testing options, gathering customer feedback, or generating valuable insights.
To understand whether certain functionalities would be fit for your UX research practice and design process, make sure you have a clear outline of your current and upcoming research needs, the type of research (generative vs. evaluative) that will be conducted, and how you will employ the different UX research methods.
For example, if your next product development stage and research needs involve collecting in-depth qualitative data, you'll likely conduct generative, moderated interviews. You'll likely want features like AI transcription, AI-driven sentiment analysis and so forth.
2. Integration
Consider how well the tool integrates with your existing tech stack. Seamless integration with platforms like your project management software, design tools, or CRM system can streamline your workflow and ensure smooth data transfer. Integrations could reduce manual data entry and allows for a more cohesive user research process.
3. Usability
Make sure to run the tool with the team members that will be actually using the tool. The tool should be user-friendly and accessible for easy team collaboration. Implementation of AI tools is only good when the they are usable in the first place.
A steep learning curve can slow down your UX research process and reduce the overall efficiency of your team. Prioritize tools that offer intuitive interfaces, clear documentation, and strong customer support.
4. Customization
Different projects may require different approaches, so it’s important to choose tools that offer a high degree of customization. Whether it's the ability to tailor survey questions, adjust data collection methods, or tweak how you approach sensemaking, customization ensures that the tool can adapt to your unique project requirements.
5. Research Data Privacy and Security
As UX research often involves handling sensitive user data, ensuring that the tool adheres to strict privacy regulations is critical. Make sure you have a clear statement of how data is protected, anonymized, encrypted, and comply with standards like GDPR.
6. Cost
Evaluate the pricing model of the tool to ensure it fits within your budget. Some tools may offer tiered pricing based on usage, while others might require a flat fee. Consider the cost in relation to the features provided and the potential ROI from improved research outcomes.
Best practices for using AI in UX research
As AI becomes increasingly integrated into UX research workflows, it's essential to follow best practices to maximize the benefits while minimizing potential pitfalls. Here are some key guidelines to use AI tools effectively:
Balance AI with human oversight
While AI can handle data analysis, pattern recognition, and automation, it lacks the nuanced understanding that user researchers bring to the table. Interpret AI-generated analysis with human expertise to validate that the insights accurately reflect user emotions and behaviors.
Just as triangulating data is essential in research, it's important to incorporate human-driven insights from qualitative user interviews and observations for a well-rounded understanding of your target audience.
Validate AI-generated insights
When using AI for data analysis, we recommend treating its results with caution and having a human review the details. While AI can significantly speed up the analysis process, it should be seen as a supplementary tool, not the primary driver of your data analysis.
- Always cross-check AI-generated insights with human analysis or other data sources. This ensures that the insights are not only accurate but also actionable.
- Use AI outputs as a starting point for further exploration rather than definitive conclusions. Continuously refine and validate insights through additional user research methods.
Use AI for what it does best
AI is not the best tool to process your large qualitative research data collected from in-depth interviews and prototype testing. AI will not accurately capture the nuance or context of a conversation. Instead, leverage AI to automate repetitive or time-consuming tasks, such as data cleaning, transcription, or initial data analysis.
AI could also be used for generating ideas for inspiration or as second eye to skim through large volumes of data to identify trends or patterns that may not be immediately apparent through manual analysis.
Ensure data privacy and ethical considerations
One of the major pitfalls for using publicly available AI tools for UX research is that you are handling participant data. Make sure that your AI tool complies with data protection laws, such as GDPR or CCPA. Anonymize user data where possible and ensure that any data processed by AI tools is securely handled.
Moreover, AI systems can inherit biases from the data they are trained on. Be vigilant about potential biases in your AI tools and take proactive steps to mitigate them, such as using diverse datasets and regularly reviewing AI outcomes for fairness.
Potential pitfalls and how to avoid them
Use of AI tool comes with potential pitfalls that can impact the quality of your insights if not carefully managed. To avoid these pitfalls, it’s essential to maintain a balance between AI and human input in your research and design
process. Use AI to handle repetitive tasks and process large amounts of data quickly, but always apply human expertise for interpretation, validation, and strategic decision-making.
Relying too much on AI
While AI can provide valuable assistance, over-relying on it can be detrimental depending on how it is used. Especially when it comes to data analysis, it may lead to misrepresentation or false insights from data.
Use AI to handle repetitive and data-intensive tasks, but rely on human researchers for interpreting in-depth analysis and making actionable recommendations.
Always make sure to validate AI-generated insights with human judgment to ensure they are sound. Combining human insights with AI tools improve your research findings become more robust, integrating data like quotes and visuals for a comprehensive analysis.
Potential for biased data
Another pitfall is the risk of biased data. AI systems are only as good as the data they are trained on, and if that data contains biases, those biases can be reflected in the AI’s output. This can skew research results and lead to flawed conclusions.
To mitigate this, it’s important to regularly review and update the data your AI tools use and actively involve manual inspection to validate the outcomes.
Go through user feedback to validate AI-generated results
Using artificial intelligence to summarize data can often result in vague summaries that lack actionable insights. While AI can quickly process large volumes of information, it may overlook nuances and context-specific details, which are ultimate keys that resonate most with your stakeholders.
This can be addressed by using AI tools as a starting point and then applying human analysis to refine and contextualize the results.
What is the best AI tools for research?
While we've explored a list of user research tools and AI tools, there is no one-size-fits-all solution, as the UX research process is inherently complex. However, certain steps can be automated to save significant time and resources, leading to quicker research turnaround and improved ROI.
As you explore different tools, consider starting with a trial or demo version of a tool to see how it integrates with your existing processes.
If you are looking for AI powered research tools for your user research process, consider looking into Hubble. Hubble streamlines your research with various testing options, such as in-product surveys, usability testing, prototype testing, along with high-quality participant recruiting.
It provides advanced heatmaps and visualization to easily make sense of study results, and AI-generated summaries to initialize your qualitative data analysis process.
If you are interested in exploring other UX research tools, we recommend some of the articles below:
- To learn more about otherUserTesting alternatives, check out 13 best UserTesting alternatives.
- To see our curated list of survey tools, see the best survey tools for research in 2025.
- To learn more about top UX research tools, please see the best user research tools in 2025.
- To see our curated list of UX testing tools, see the best usability testing tools in 2025.